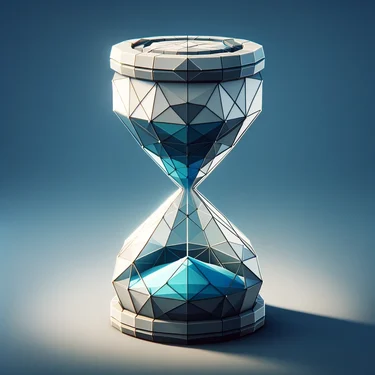
Each man's death diminishes me,
For I am involved in mankind.
Therefore, send not to know
For whom the bell tolls,
It tolls for thee.
from For Whom the Bell Tolls by John Donne
Life Expectancy Predictor
Maybe it’s curiosity that brought you here, or maybe you are concerned about a loved one or even yourself. I don’t need to know. The main thing is that you’re here, so let’s quickly talk about what this tool is and what it is not.
This is for research purposes only. It should be something that provides you with information that you can then validate or dispute through conversations with your doctor and through your own research. To see how this tool was created and the data sources that it uses, please scroll down to the bottom of the page.
Data Sources
Demographic Studies: Analyzing demographic data can provide insights into life expectancy trends based on factors like age, gender, race, ethnicity, and geographic location.
Longitudinal Studies: Long-term studies tracking individuals over their lifetimes can offer valuable data on lifestyle choices, medical history, and environmental factors affecting longevity.
Epidemiological Studies: These studies investigate patterns, causes, and effects of health and disease conditions in defined populations, helping identify risk factors associated with mortality.
Medical Records and Health Surveys: Accessing medical records and health surveys can provide information on pre-existing conditions, disease prevalence, treatment history, and lifestyle habits that impact life expectancy.
Genetic Studies: Genetic factors play a significant role in determining longevity. Genetic studies can identify genetic variants associated with lifespan and susceptibility to age-related diseases.
Behavioral Studies: Understanding behaviors such as smoking, diet, exercise, alcohol consumption, and drug use is crucial for predicting life expectancy. Behavioral studies can provide data on how these factors affect health outcomes.
Environmental and Socioeconomic Data: Factors like pollution levels, access to healthcare, socioeconomic status, education level, and employment opportunities can influence life expectancy. Incorporating environmental and socioeconomic data enhances the accuracy of predictions.
Machine Learning Models: Utilizing machine learning algorithms can help analyze complex datasets and identify patterns that contribute to life expectancy prediction. These models can integrate multiple variables and adjust predictions based on new data.
Public Health Databases: Accessing public health databases maintained by government agencies or research institutions can provide standardized data on mortality rates, causes of death, and population demographics.
Expert Consensus and Guidelines: Consulting medical professionals, epidemiologists, and researchers can provide valuable insights into selecting relevant variables, validating models, and interpreting results accurately.