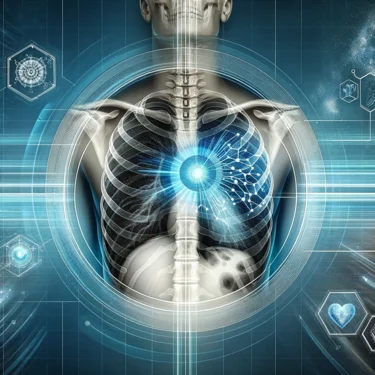
AI Revolution in COVID-19 Diagnosis
Deep neural networks have produced state of the art breakthroughs in the most difficult areas of artificial intelligence and have reached mainstream appeal through the release of Chat GPT, Stable Diffusion, Snapchat filters, and similar tools for the broader public. And, it appears they’ve done it again. This time in the medical arena. In an unprecedented advancement in medical technology, a team of researchers from the University of Technology Sydney (UTS), led by Professor Amir H Gandomi, has unveiled a groundbreaking Artificial Intelligence (AI) system capable of detecting COVID-19 in chest X-rays with astounding accuracy. This development, recently published in Nature Scientific Reports, represents a significant stride in the ongoing battle against the COVID-19 pandemic, addressing critical challenges in timely and accurate diagnosis.
Breaking New Ground in COVID-19 Detection
The novel AI system employs a deep learning-based algorithm known as Custom Convolutional Neural Network (Custom-CNN). This sophisticated tool differentiates between COVID-19, normal cases, and pneumonia with more than 98% accuracy, offering a rapid and reliable alternative to the conventional real-time polymerase chain reaction (PCR) testing. PCR tests, though widely used, are hampered by limitations such as cost, time constraints, and a tendency to produce false negatives.
Convolutional Neural Networks (CNNs)
The Custom-CNN model, specifically engineered for COVID-19 diagnosis, marks a departure from traditional diagnostic methods. It leverages deep learning to analyze chest X-rays, bypassing the need for manual biomarker identification and significantly reducing the time and potential errors associated with human examination. This AI system not only speeds up the diagnostic process but also enhances its precision, making it a formidable tool in the early detection of COVID-19 infection.
Deep Dive into CNNs
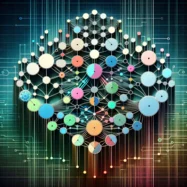
Convolutional Neural Networks (CNNs) are a specialized type of neural network used primarily for processing data with a grid-like topology, such as images. CNNs are designed to automatically and adaptively learn spatial hierarchies of features through a process known as convolution. The fundamental building blocks of CNNs are convolutional layers, pooling layers, and fully connected layers. In a convolutional layer, small regions of the input image are scanned by filters or kernels. These filters detect spatial patterns such as edges, textures, or more complex patterns in higher layers. Pooling layers then reduce the spatial dimensions (width and height) of the input volume for the next convolutional layer, effectively reducing the number of parameters and computation in the network. This operation also helps with making the representation more invariant to small translations in the input. Finally, fully connected layers combine all the learned features from previous layers across the entire image to classify the image into different categories, depending on the task at hand. CNNs are particularly powerful in image recognition and classification tasks and have been instrumental in driving advances in computer vision.
Addressing Global Healthcare Challenges
One of the critical advantages of this AI system is its potential application in regions experiencing high COVID-19 caseloads and facing a scarcity of radiologists. Chest X-rays, being portable and widely available with lower exposure to ionizing radiation than CT scans, make for an ideal medium for AI analysis. This technology could alleviate the strain on healthcare systems by providing a swift and efficient diagnostic method, particularly in countries where access to advanced medical resources is limited.
Transforming COVID-19 Management
The implications of this AI breakthrough extend far beyond mere diagnosis. Fast and accurate identification of COVID-19 cases ensures that patients receive appropriate treatment, including timely administration of antivirals, which are most effective in the initial days of symptom onset. Furthermore, it empowers individuals to self-isolate promptly, curtailing the spread of the virus and mitigating the broader impact on public health and the global economy.
Future Directions and Ethical Considerations
As we embrace this AI-driven approach, it’s crucial to consider the ethical aspects of integrating AI into healthcare. The balance between human expertise and AI support must be carefully managed to ensure that AI augments rather than replaces the critical judgment of medical professionals. Moreover, data privacy and the security of patient information in AI systems remain paramount concerns that need to be addressed as this technology evolves.
The Road Ahead
The success of this deep learning model in COVID-19 diagnosis is just the tip of the iceberg. This breakthrough paves the way for further exploration and application of AI in various areas of medicine, potentially transforming how we approach disease diagnosis and management. As AI continues to evolve, it holds the promise of revolutionizing healthcare, offering more efficient, accurate, and accessible medical solutions.
Conclusion
Professor Gandomi’s team in Sydney has not only contributed significantly to the fight against COVID-19 but has also set a precedent for the use of AI in medical diagnostics. This innovation stands as a testament to the power of technology in enhancing our response to global health crises. As we continue to navigate the challenges of the COVID-19 pandemic, such advancements in AI provide a beacon of hope, signifying a new era in healthcare where technology and human expertise converge to combat the most pressing medical challenges of our time.